MSPB: a longitudinal multi-sensor dataset with phenotypic trait measurements from honeybees
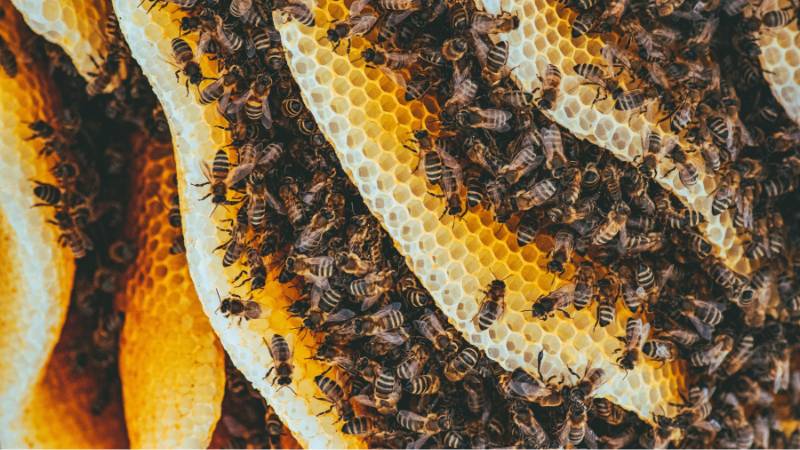
Honeybees (Apis mellifera) are vital for pollination and biodiversity, but beekeepers face challenges in monitoring their colonies, traditionally relying on manual inspections that are time-consuming and disruptive. With rising colony losses due to stressors like pesticides and climate change, continuous monitoring is essential. To address this, automated beehive monitoring systems have been developed using sensors to track environmental conditions and colony status. Data from sensors such as temperature, humidity, and acoustics are fed into machine learning (ML) models to predict hive conditions, such as winter survival and queen activity. However, large amounts of data are needed for accurate ML predictions. To support this, the Multi-modal Sensor dataset with Phenotypic trait measurements from honeybees (MSPB) was created, featuring data from 53 hives over one year in Québec, Canada. This dataset includes audio,
temperature, and humidity data, along with phenotypic traits like honey yield, brood counts, and Varroa mite infestation, facilitating advanced ML-driven hive monitoring and analysis. Read the full article on Nature.